如何提升 ETF 期权隐含波动率和希腊值的计算速度?
期权的隐含波动率可以反应市场对未来的预期,通常使用牛顿法和二分法来计算。这两种方法都需要频繁迭代,且迭代次数不能确定,核心代码无法向量化,因此只能通过循环来逼近求解。这就导致在期权相关计算中,隐含波动率往往容易成为性能的瓶颈。
DolphinDB 的计算逻辑使用脚本语言编写,但底层调用的是 C++ 代码,存在脚本解释的过程。为了提高脚本的执行效率,DolphinDB 从 1.01 版本开始支持即时编译(JIT)功能,特别适合于无法使用向量化运算但又对运行速度有极高要求的场景。
本教程将基于客户的实际需求,以二分法计算 ETF 期权的隐含波动率及希腊值为例,为大家示范如何使用 DolphinDB 的 JIT 功能给计算过程加速,并与 C++ 原生代码进行了计算性能对比测试,结果表明 DolphinDB 脚本计算耗时为 C++ 原生代码的1.5倍。
1. 数据表结构
1.1 期权日频数据表
字段 |
字段类型 |
含义 |
tradedate |
DATE |
交易日期 |
sym |
SYMBOL |
标的代码 |
codes |
SYMBOL |
期权合约代码 |
closeprice |
DOUBLE |
日收盘价格 |
etf |
SYMBOL |
期权合成价格的两个合约代码 |
etfprice |
DOUBLE |
期权合成价格 |
字符串字段使用 SYMBOL 类型和 STRING 类型存储的差异,参考: 数据类型 — DolphinDB 2.0 文档的字符串部分内容。
期权日频数据表在 DolphinDB 中存储时,建议在时间维度按年分区即可,创建库表的代码如下:
login("admin", "123456")
dbName = "dfs://optionPrice"
tbName = "optionPrice"
if(existsDatabase(dbName)){
dropDatabase(dbName)
}
db = database(dbName, RANGE, date(datetimeAdd(2000.01M,0..50*12,'M')))
colNames = `tradedate`sym`codes`closeprice`etf`etfprice
colTypes = [DATE, SYMBOL, SYMBOL, DOUBLE, SYMBOL, DOUBLE]
schemaTable = table(1:0, colNames, colTypes)
db.createPartitionedTable(table=schemaTable, tableName=tbName, partitionColumns=`tradedate)
读取 50 ETF 日频数据,代码如下:
data = select * from loadTable("dfs://optionPrice", "optionPrice") where sym =`510050
data 是表变量,具体数据内容如下图所示:

通过 panel 函数对 data 进行透视操作,将窄表数据展成矩阵,生成期权日收盘价矩阵,代码如下:
closPriceWideMatrix = panel(data.codes, data.tradeDate, data.closePrice)
closPriceWideMatrix 是矩阵变量,具体数据内容如下图所示:
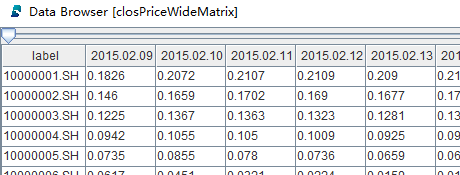
通过 panel 函数对 data 进行透视操作,将窄表数据展成矩阵,生成期权合成价格矩阵,代码如下:
etfPriceWideMatrix = panel(data.codes, data.tradeDate, data.etfprice)
etfPriceWideMatrix 是矩阵变量,具体数据内容如下图所示:
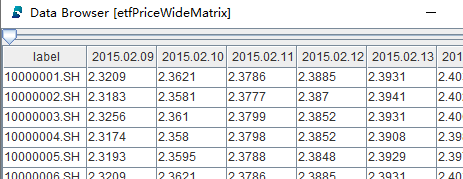
1.2 期权信息
字段 |
字段类型 |
含义 |
code |
STRING |
期权合约代码 |
name |
STRING |
期权合约名称 |
exemode |
INT |
期权类型(认购认沽类型) |
exeprice |
DOUBLE |
行权价 |
startdate |
DATE |
开始日期 |
lastdate |
DATE |
结束日期 |
sym |
SYMBOL |
标的代码 |
exeratio |
DOUBLE |
乘数 |
exeprice2 |
DOUBLE |
分红后的行权价 |
dividenddate |
DATE |
分红日 |
tradecode |
STRING |
交易代码 |
期权信息数据在 DolphinDB 中存储时,建议在证券代码维度按值分区即可,创建库表的代码如下:
login("admin", "123456")
dbName = "dfs://optionInfo"
tbName = "optionInfo"
if(existsDatabase(dbName)){
dropDatabase(dbName)
}
db = database(dbName, VALUE, `510050`510300)
colNames = `code`name`exemode`exeprice`startdate`lastdate`sym`exeratio`exeprice2`dividenddate`tradecode
colTypes = [STRING, STRING, INT, DOUBLE, DATE, DATE, SYMBOL, DOUBLE, DOUBLE, DATE, STRING]
schemaTable = table(1:0, colNames, colTypes)
db.createPartitionedTable(table=schemaTable, tableName=tbName, partitionColumns=`sym)
读取数据:
contractInfo = select * from loadTable("dfs://optionInfo", "optionInfo") where sym =`510050
contractInfo 是表变量,具体数据内容如下图所示:

1.3 交易日历
字段 |
字段类型 |
含义 |
tradedate |
DATE |
交易日期 |
交易日历存放在单列 csv 文件中,可以使用 DolphinDB 的 loadText 函数接口直接读取:
//交易日历csv文件路径
tradingDatesAbsoluteFilename = "/hdd/hdd9/tutorials/jitAccelerated/tradedate.csv"
startDate = 2015.02.01
endDate = 2022.03.01
//读取csv文件
allTradingDates = loadText(tradingDatesAbsoluteFilename)
//生成交易日向量
tradingDates = exec tradedate from allTradingDates where tradedate<endDate and tradedate >startDate
allTradingDates 是表变量,可以使用 exec 函数将表中的某一列转成向量变量,tradingDates 是向量变量。
2. 计算函数代码开发
2.1 隐含波动率
DolphinDB 脚本语言需要先解释再执行,计算密集的代码如果不能向量化,在脚本层面使用 while 和 for 循环以及条件分支,就会比较耗时。期权隐含波动率计算的步骤,由于使用了上下限值循环逼近的二分法,正是需要 JIT 加速的计算类型。以下就是二分法逼近隐含波动率的代码:
@jit
def calculateD1JIT(etfTodayPrice, KPrice, r, dayRatio, HLMean){
skRatio = etfTodayPrice / KPrice
denominator = HLMean * sqrt(dayRatio)
result = (log(skRatio) + (r + 0.5 * pow(HLMean, 2)) * dayRatio) / denominator
return result
}
@jit
def calculatePriceJIT(etfTodayPrice, KPrice , r , dayRatio , HLMean , CPMode){
testResult = 0.0
if (HLMean <= 0){
testResult = CPMode * (etfTodayPrice - KPrice)
if(testResult<0){
return 0.0
}
return testResult
}
d1 = calculateD1JIT(etfTodayPrice, KPrice, r, dayRatio, HLMean)
d2 = d1 - HLMean * sqrt(dayRatio)
price = CPMode * (etfTodayPrice * cdfNormal(0, 1, CPMode * d1) - KPrice * cdfNormal(0, 1, CPMode * d2) * exp(-r * dayRatio))
return price
}
@jit
def calculateImpvJIT(optionTodayClose, etfTodayPrice, KPrice, r, dayRatio, CPMode){
v = 0.0
high = 2.0
low = 0.0
do{
if ((high - low) <= 0.00001){
break
}
HLMean = (high + low) / 2.0
if (calculatePriceJIT(etfTodayPrice, KPrice, r, dayRatio, HLMean, CPMode) > optionTodayClose){
high = HLMean
}
else{
low = HLMean
}
}
while(true)
v = (high + low) / 2.0
return v
}
def calculateImpv(optionTodayClose, etfTodayPrice, KPrice, r, dayRatio, CPMode){
originalShape = optionTodayClose.shape()
optionTodayClose_vec = optionTodayClose.reshape()
etfTodayPrice_vec = etfTodayPrice.reshape()
KPrice_vec = KPrice.reshape()
dayRatio_vec = dayRatio.reshape()
CPMode_vec = CPMode.reshape()
impvTmp = each(calculateImpvJIT, optionTodayClose_vec, etfTodayPrice_vec, KPrice_vec, r, dayRatio_vec, CPMode_vec)
impv = impvTmp.reshape(originalShape)
return impv
}
calculateImpvJIT 是计算隐含波动的核心代码,其入参 optionTodayClose, etfTodayPrice, KPrice, r, dayRatio, CPMode 都是标量对象,其调用的 calculatePriceJIT 函数和 calculateD1JIT 函数都通过 @jit 装饰器的方式封装成JIT函数,以达到加速计算的目的。
calculateImpv 是计算隐含波动的最终调用函数,其入参 optionTodayClose, etfTodayPrice, KPrice, dayRatio, CPMode 都是矩阵对象,其主要作用是把输入和输出进行矩阵和向量的转换,以适不同函数的入参和输出。在后面 delta, gamma, vega, theta 计算时,也会用到这些矩阵入参,这里以 2015年2月16日的 50ETF 为例进行展示。
optionTodayClose

etfTodayPrice

KPrice

dayRatio

CPMode

2.2 delta
delta 表示期权价格对标的资产价格的变动率,即标的资产价格每变动一个单位,期权价格产生的变化。
delta 的计算可以方便地实现向量化计算,所以不需要调用 JIT 功能,其代码如下:
def calculateD1(etfTodayPrice, KPrice, r, dayRatio, HLMean){
skRatio = etfTodayPrice / KPrice
denominator = HLMean * sqrt(dayRatio)
result = (log(skRatio) + (r + 0.5 * pow(HLMean, 2)) * dayRatio) / denominator
return result
}
def cdfNormalMatrix(mean, stdev, X){
originalShape = X.shape()
X_vec = X.reshape()
result = cdfNormal(mean, stdev, X_vec)
return result.reshape(originalShape)
}
def calculateDelta(etfTodayPrice, KPrice, r, dayRatio, impvMatrix, CPMode){
delta = iif(
impvMatrix <= 0,
0,
0.01*etfTodayPrice*CPMode*cdfNormalMatrix(0, 1, CPMode * calculateD1(etfTodayPrice, KPrice, r, dayRatio, impvMatrix))
)
return delta
}
calculateDelta 是计算 delta 的最终调用函数,其入参 etfTodayPrice, KPrice, dayRatio, impvMatrix, CPMode 都是矩阵对象。
2.3 gamma
gamma 表示 delta 对于标的资产价格的变动率,即标的资产价格每变动一个单位,delta 值产生的变化。
gamma 的计算可以方便地实现向量化计算,所以不需要调用 JIT 功能,其代码如下:
def normpdf(x){
return exp(-pow(x, 2)/2.0)/sqrt(2*pi)
}
def calculateD1(etfTodayPrice, KPrice, r, dayRatio, HLMean){
skRatio = etfTodayPrice / KPrice
denominator = HLMean * sqrt(dayRatio)
result = (log(skRatio) + (r + 0.5 * pow(HLMean, 2)) * dayRatio) / denominator
return result
}
def calculateGamma(etfTodayPrice, KPrice, r, dayRatio, impvMatrix){
gamma = iif(
impvMatrix <= 0,
0,
(normpdf(calculateD1(etfTodayPrice, KPrice, r, dayRatio, impvMatrix)) \ (etfTodayPrice * impvMatrix * sqrt(dayRatio))) * pow(etfTodayPrice, 2) * 0.0001
)
return gamma
}
calculateGamma 是计算 gamma 的最终调用函数,其入参 etfTodayPrice, KPrice, dayRatio, impvMatrix 都是矩阵对象。
2.4 vega
vega 表示波动率单位变动对期权价格产生的变化。
vega 的计算可以方便地实现向量化计算,所以不需要调用 JIT 功能,其代码如下:
def normpdf(x){
return exp(-pow(x, 2)/2.0)/sqrt(2*pi)
}
def calculateD1(etfTodayPrice, KPrice, r, dayRatio, HLMean){
skRatio = etfTodayPrice / KPrice
denominator = HLMean * sqrt(dayRatio)
result = (log(skRatio) + (r + 0.5 * pow(HLMean, 2)) * dayRatio) / denominator
return result
}
def calculateVega(etfTodayPrice, KPrice, r, dayRatio, impvMatrix){
vega = iif(
impvMatrix <= 0,
0,
etfTodayPrice * normpdf(calculateD1(etfTodayPrice, KPrice, r, dayRatio, impvMatrix)) * sqrt(dayRatio)
)
return vega \ 100.0
}
calculateVega 是计算 vega 的最终调用函数,其入参 etfTodayPrice, KPrice, dayRatio, impvMatrix 都是矩阵对象。
2.5 theta
theta 表示时间流逝对期权价格产生的变化,即每减少一天,期权价格的变化值。
theta 的计算可以方便地实现向量化计算,所以不需要调用 JIT 功能,其代码如下:
def calculateD1(etfTodayPrice, KPrice, r, dayRatio, HLMean){
skRatio = etfTodayPrice / KPrice
denominator = HLMean * sqrt(dayRatio)
result = (log(skRatio) + (r + 0.5 * pow(HLMean, 2)) * dayRatio) / denominator
return result
}
def normpdf(x){
return exp(-pow(x, 2)/2.0)/sqrt(2*pi)
}
def cdfNormalMatrix(mean, stdev, X){
originalShape = X.shape()
X_vec = X.reshape()
result = cdfNormal(mean, stdev, X_vec)
return result.reshape(originalShape)
}
def calculateTheta(etfTodayPrice, KPrice, r, dayRatio, impvMatrix, CPMode){
annualDays = 365
d1 = calculateD1(etfTodayPrice, KPrice, r, dayRatio, impvMatrix)
d2 = d1 - impvMatrix * sqrt(dayRatio)
theta = (-etfTodayPrice * normpdf(d1) * impvMatrix \ (2 * sqrt(dayRatio)) - CPMode * r * KPrice * exp(-r * dayRatio) *cdfNormalMatrix(0, 1, CPMode * d2)) \ annualDays
result = iif(impvMatrix<= 0, 0, theta)
return result
}
calculateTheta 是计算 theta 的最终调用函数,其入参 etfTodayPrice, KPrice, dayRatio, impvMatrix, CPMode 都是矩阵对象。
2.6 单日计算函数
开发完最核心的计算函数后,可以自定义一个单日计算函数,计算指定日期的隐含波动率和希腊值,其代码如下:
def calculateOneDayGreek(closPriceWideMatrix, etfPriceWideMatrix, contractInfo, targetDate){
targetDate_vec = [targetDate]
r = 0
optionTodayClose = getTargetDayOptionClose(closPriceWideMatrix, targetDate_vec)
validContractsToday = optionTodayClose.columnNames()
etfTodayPrice = getTargetDayEtfPrice(etfPriceWideMatrix, targetDate_vec)
KPrice, dayRatio, CPMode = getTargetDayContractInfo(contractInfo, validContractsToday, targetDate_vec)
impvMatrix = calculateImpv(optionTodayClose, etfTodayPrice, KPrice, r, dayRatio, CPMode)
deltaMatrix = calculateDelta(etfTodayPrice, KPrice, r, dayRatio, impvMatrix, CPMode)\(etfTodayPrice*0.01)
gammaMatrix = calculateGamma(etfTodayPrice, KPrice, r, dayRatio, impvMatrix)\(pow(etfTodayPrice, 2) * 0.0001)
vegaMatrix = calculateVega(etfTodayPrice, KPrice, r, dayRatio, impvMatrix)
thetaMatrix = calculateTheta(etfTodayPrice, KPrice, r, dayRatio, impvMatrix, CPMode)
todayTable = table(validContractsToday as optionID, impvMatrix.reshape() as impv, deltaMatrix.reshape() as delta, gammaMatrix.reshape() as gamma, vegaMatrix.reshape() as vega, thetaMatrix.reshape() as theta)
todayTable["tradingDate"] = targetDate
todayTable.reorderColumns!(["optionID", "tradingDate"])
return todayTable
}
calculateOneDayGreek 的入参 closPriceWideMatrix, etfPriceWideMatrix 是矩阵对象(参考第1章节的读取数据),contractInfo 是表对象(参考第1章节的读取数据),targetDate 是标量对象。
calculateOneDayGreek 函数还调用了 getTargetDayOptionClose 函数, getTargetDayEtfPrice 函数和getTargetDayContractInfo 函数,调用的目的是从全量数据中获取计算当日的有效信息,代码如下:
/*
* 按合约和交易日在期权日频收盘价矩阵中寻找对应价格
*/
def getTargetDayOptionClose(closPriceWideMatrix, targetDate){
colNum = closPriceWideMatrix.colNames().find(targetDate)
return closPriceWideMatrix[colNum].transpose().dropna(byRow = false)
}
/*
* 按合约和交易日在期权合成期货价格矩阵中寻找对应价格
*/
def getTargetDayEtfPrice(etfPriceWideMatrix, targetDate){
colNum = etfPriceWideMatrix.colNames().find(targetDate)
return etfPriceWideMatrix[colNum].transpose().dropna(byRow = false)
}
/*
* 根据合约和交易日在期权信息表中寻找 KPrice, dayRatio, CPMode
*/
def getTargetDayContractInfo(contractInfo, validContractsToday, targetDate){
targetContractInfo = select code, exemode, exeprice, lastdate, exeprice2, dividenddate, targetDate[0] as tradingDate from contractInfo where Code in validContractsToday
KPrice = exec iif(tradingDate<dividenddate, exeprice2, exeprice) from targetContractInfo pivot by tradingDate, code
dayRatio = exec (lastdate-tradingDate)\365.0 from targetContractInfo pivot by tradingDate, Code
CPMode = exec exemode from targetContractInfo pivot by tradingDate, Code
return KPrice, dayRatio, CPMode
}
calculateOneDayGreek 函数的具体使用方法会在下一章说明。
2.7 多日并行计算函数
自定义单日计算函数后,可以再自定义一个多日并行计算函数,其代码如下:
def calculateAll(closPriceWideMatrix, etfPriceWideMatrix, contractInfo, tradingDates, mutable result){
calculator = partial(calculateOneDayGreek, closPriceWideMatrix, etfPriceWideMatrix, contractInfo)
timer{
allResult = ploop(calculator, tradingDates)
}
for(oneDayResult in allResult){
append!(result, oneDayResult)
}
}
calculateAll 是自定义的多日并行计算函数,主要用到了 DolphinDB 内置的 partial 部分应用函数和 ploop 并行计算函数,直接传入要并行的函数和入参,不必像其他语言一样先定义线程池/进程池。calculateAll 函数的具体使用方法会在下一章说明。
3. 计算性能测试
测试的交易日范围从2015年2月到2022年3月,实际交易日1729天。测试的期权品种是 50 ETF,证券代码为510050,涉及期权合约共3124个。
3.1 测试环境
CPU 类型:Intel(R) Xeon(R) Silver 4216 CPU @ 2.10GHz
逻辑 CPU 总数:8
内存:64GB
OS:64位 CentOS Linux 7 (Core)
DolphinDB server 版本:2.00.8 JIT
3.2 单日计算性能测试
单线程单日计算性能测试代码如下:
//定义单日性能测试函数
def testOneDayPerformance(closPriceWideMatrix, etfPriceWideMatrix, contractInfo, targetDate){
targetDate_vec = [targetDate]
r = 0
optionTodayClose = getTargetDayOptionClose(closPriceWideMatrix, targetDate_vec)
validContractsToday = optionTodayClose.columnNames()
etfTodayPrice = getTargetDayEtfPrice(etfPriceWideMatrix, targetDate_vec)
KPrice, dayRatio, CPMode = getTargetDayContractInfo(contractInfo, validContractsToday, targetDate_vec)
timer{
impvMatrix = calculateImpv(optionTodayClose, etfTodayPrice, KPrice, r, dayRatio, CPMode)
deltaMatrix = calculateDelta(etfTodayPrice, KPrice, r, dayRatio, impvMatrix, CPMode)\(etfTodayPrice*0.01)
gammaMatrix = calculateGamma(etfTodayPrice, KPrice, r, dayRatio, impvMatrix)\(pow(etfTodayPrice, 2) * 0.0001)
vegaMatrix = calculateVega(etfTodayPrice, KPrice, r, dayRatio, impvMatrix)
thetaMatrix = calculateTheta(etfTodayPrice, KPrice, r, dayRatio, impvMatrix, CPMode)
}
todayTable = table(validContractsToday as optionID, impvMatrix.reshape() as impv, deltaMatrix.reshape() as delta, gammaMatrix.reshape() as gamma, vegaMatrix.reshape() as vega, thetaMatrix.reshape() as theta)
todayTable["tradingDate"] = targetDate
todayTable.reorderColumns!(["optionID", "tradingDate"])
return todayTable
}
//执行单日性能测试函数
oneDay = testOneDayPerformance(closPriceWideMatrix, etfPriceWideMatrix, contractInfo, 2022.02.28)
测试结果如下:
计算日期为 2022年2月28日
测试的期权品种是 50 ETF,涉及期权合约共136个
DolphinDB 脚本计算总耗时为2.1 ms
C++ 原生代码计算总耗时为1.02 ms

3.3 多日并行计算性能测试
多日并行计算性能测试代码如下:
//创建存储计算结果的表变量
result = table(
array(SYMBOL, 0) as optionID,
array(DATE, 0) as tradingDate,
array(DOUBLE, 0) as impv,
array(DOUBLE, 0) as delta,
array(DOUBLE, 0) as gamma,
array(DOUBLE, 0) as vega,
array(DOUBLE, 0) as theta
)
//执行多日并行计算函数
calculateAll(closPriceWideMatrix, etfPriceWideMatrix, contractInfo, tradingDates, result)
测试结果如下:
计算日期为 2015年2月到2022年3月
测试的期权品种是 50 ETF,涉及期权合约共3124个
计算的并行度为8,测试环境的8个 CPU 满负荷运行
DolphinDB 脚本计算总耗时为300 ms
C++ 原生代码计算总耗时为200 ms
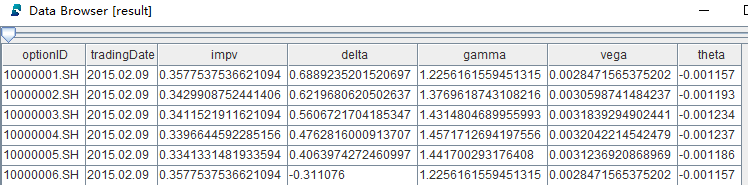
计算过程中的 CPU 使用率:
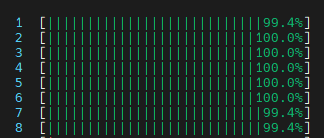
4. 总结
本教程中期权隐含波动率的计算使用了 JIT 功能提速,其余希腊值的计算使用了向量化计算。我们测试了2015年2月到2022年3月 50 ETF 所有期权合约的隐含波动率和希腊值的计算性能,在8个 CPU 满负荷运行下,DolphinDB 脚本计算总耗时为300 ms,C++ 原生代码计算总耗时为200 ms,耗时相差50%左右。
关于 DolphinDB JIT 的更多详细特性,可以参考 DolphinDB JIT教程。
通过 DolphinDB 下载连接下载 DolphinDB server 进行测试的时候,必须选择包含 JIT 功能的安装包,如下图所示:
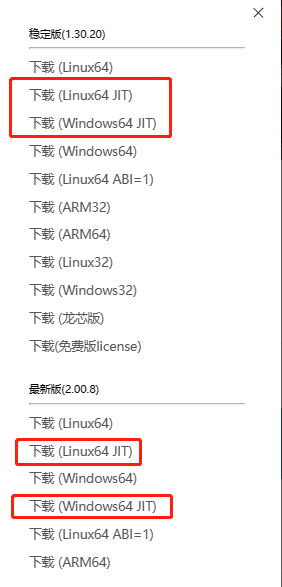
附录
- DolphinDB 流计算应用:引擎级联监测门禁异常状态
- DolphinDB 数据迁移与再平衡
- DolphinDB 机器学习在物联网行业的应用:实时数据异常率预警
- 如何提升 ETF 期权隐含波动率和希腊值的计算速度?
- 如何用 Prometheus 和 Grafana 实现集群的监控预警
- DolphinDB 分布式表数据更新原理和性能介绍
- 时序数据库 DolphinDB 线程简介
- 中高频多因子库存储最佳实践
- 如何高效处理面板数据
- 性能提升30倍丨基于 DolphinDB 的 mytt 指标库实现
- 如何使用VS2017编译DolphinDB C API动态库
- DolphinDB 函数化编程案例教程
- DolphinDB 版本兼容性标准
- 更强大、更灵活、更全面丨一文搞懂DolphinDB窗口计算
- 从一次 SQL 查询的全过程看 DolphinDB 的线程模型
- 支持事务,还是不支持事务?这是一个问题
- DolphinDB 用户社区「AskDolphinDB」正式上线!!
- DolphinDB案例分享丨帆软报表软件如何连接DolphinDB数据源
- 干货丨时序数据库DolphinDB与Spark的性能对比测试报告
- 测试报告丨DolphinDB与Elasticserach在金融数据集上的性能对比测试